目的
RISC-VのSBCであるVisionFive 2でTensorFlow LiteのXNNPACK
delegateを実行してみる。
RISC-V CPUでTensorFlow Lite。
環境
- VisionFive 2 Debian Image 202302 Released
- OpenCV 4.7.0(build from source)
- TensorFlow Lite v2.12 Python Interpreter(build from source)
つまづきポイント
-
RISC-V CPU向けのTensorFlow Lite
Python Interpreterは提供されていない。
このため、ソースからビルド(CMakeを使う)が必要。
ただし、バグがありビルドできないためパッチをあてて対処。
構築
今回はOpenCVを使ってUSBカメラのキャプチャした画像をEfficientDet
Liteを使って物体検出してみる。
なお、動作させてみるPythonコードは自分のリポジトリを使用。
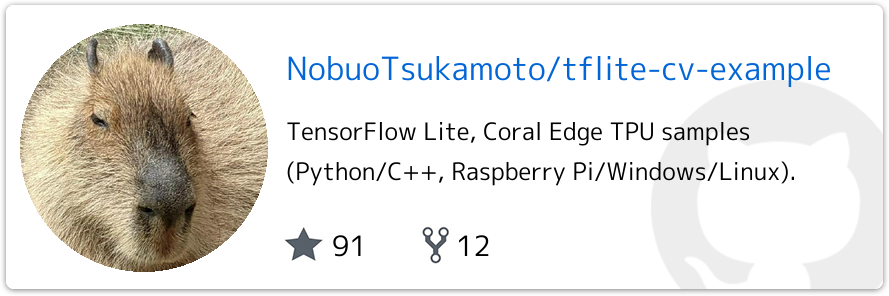
OpenCVのビルド&インストール
まず、OpenCVをソースビルドし、インストールする。
これは、自分の前回のブログを参照。
TensorFlow Lite(Python Interpreter)のビルド&インストール
TensorFlow v2.12.0でビルドを行う。
CMakeでビルドする必要があるが、r2.12ブランチではバグがありビルドできない。。。(masterブランチでは修正済みの模様)
このため、tensorflow/lite/CMakeLists.txtを修正する。
自分がやっているTensorFlow
Liteのyoctoのレシピであるmeta-tensorflow-liteにあるパッチをあてて対処。
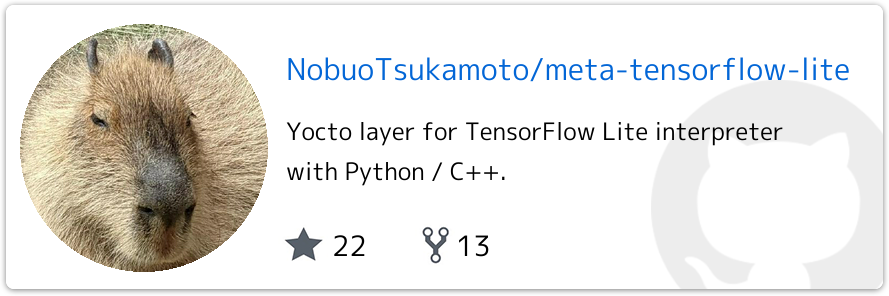
TensorFlowのリポジトリをcloneし、パッチをあてて、ビルドしてみる。
ビルドはおおよそ30分ぐらい?
$ sudo apt install swig libjpeg-dev zlib1g-dev python3-dev python3-numpy $ sudo apt install python3-pybind11 $ sudo apt install python3-setuptools $ sudo apt install python3-wheel $ sudo apt install python3-pip $ cd ~ $ git clone https://github.com/NobuoTsukamoto/meta-tensorflow-lite.git $ git clone https://github.com/tensorflow/tensorflow.git $ cd tensorflow/ $ git checkout v2.12.0 $ git apply ~/meta-tensorflow-lite/recipes-framework/tensorflow-lite/files/001-v2.12-Fix-CMAKE_Build_Error.patch $ export BUILD_NUM_JOBS=4 $ ./tensorflow/lite/tools/pip_package/build_pip_package_with_cmake.sh pip3 install /home/user/tensorflow/tensorflow/lite/tools/pip_package/gen/tflite_pip/python3/dist/tflite_runtime-2.12.0-cp310-cp310-linux_riscv64.whl
EfficientDet LiteをTensorFlow Lite Modelにエクスポート
モバイル向けの物体検出モデルであるEfficentDet LiteをTensorflow
Liteにエクスポートする。
これはホストPC(やGoogle Colaboratory)で実行する。
以前、自分が作成したエクスポート用のノートブックを利用。
エクスポートしたモデル(今回はEfficientDet-Lite0)をボードにSCPなどで転送しておく。
今回は/home/user/modelsの配下に格納。
動作確認
USBカメラを接続して動作確認してみる。
$ cd ~ $ git clone https://github.com/NobuoTsukamoto/tflite-cv-example.git $ cd tflite-cv-example/detection/python $ python3 object_detection_tflite_capture_opencv.py --model /home/user/models/efficientdet-lite0_fp32.tflite --label ../models/coco_labels.txt --thread 4
なお、コードではカメラIDを0固定で指定しているので、適宜書き換え(いつか直す)。
動作させたときの画面キャプチャ。
EfficientDet Lite0 FP32でだいたい500~600ms(4 Threads)。
💯🎉💯🎉💯🎉💯🎉💯🎉💯🎉💯🎉
— nb.o (@Nextremer_nb_o) March 17, 2023
VisionFive 2 (Debian Image 202302)
TensorFlow Lite v2.12rc1 XNNPACK delegate
Model : EfficientDet-lite0
👍👏👍👏👍👏👍👏👍👏👍👏👍👏 pic.twitter.com/XqxuTBNnr3
最後に
今回はRISC-V CPUでTensorFlow Liteをやってみた。VisionFive 2だと割とサクサク動いて感動!(たしかにラズパイ4と比べるとだけど)。
次はEdgeTPUのアクセラレターとの組み合わせかな?